- Business Operational Analytics vs. Traditional Analytics
- Why Invest in Operational Analytics: Use Cases and Advantages
- Delivering Personalized Offers
- Streamlining Agile Development
- Predictive Operational Analytics
- Customer Support
- Workforce Management
- Inventory Management
- Reducing Customer Churn
- Enhancing Risk Modeling
- Real-World Examples of Companies Using Operational Analytics
- Amazon
- Nissan
- Shell
- How to Implement Operational Analytics in Business?
- Define Clear Objectives
- Establish Relevant KPIs
- Collect and Correct Data
- Select the Right Tech Stack
- Build an Integrated Analytics Platform
- Conduct a Pilot Test
- Roll Out the System
- Monitor and Maintain
- Optimizing Business Operations Analytics: Best Practices for Success
- Align Data with Key Business Goals
- Focus on One Key Use Case Initially
- Tailor Data-Analysis Tools
- Prioritize Data-Driven Decision-Making
- Enhance Analytical Competence
- Highlight Benefits Across Teams
- Leverage External Data Sources
- Challenges and Solutions for Operational Analytics Implementation
- Data Silos
- Integration with Legacy Systems
- Resistance to Change
- Future of Operational Analytics for Businesses
- How Appinventiv Helps You Reach Operational Analytics Excellence
- FAQs
Data is the new asset in today’s digital age, prompting businesses across industries to explore innovative ways to leverage its transformative power and gain a competitive edge. This is where business operational analytics steps in. It has emerged as a game-changer for companies aiming to optimize performance, streamline operations, and make data-driven decisions.
By tapping into real-time insights from various business processes, operational data analysis enables organizations to identify inefficiencies, predict outcomes, increase profits, and adapt quickly to changes. This transformative approach not only boosts operational efficiency but also strengthens competitiveness in today’s fast-paced markets. Without custom operational analytics, many businesses often find themselves operating in the dark, relying on intuition rather than data-driven insights for critical decision-making.
Want to know more about IT operations analytics use cases, benefits, implementation strategies, and best practices? This blog is your go-to guide, helping you unlock the true power of analytics in operations and turn raw data into a strategic asset for growth. But before we delve deeper to understand the transformative power of operational analytics services, let’s first know how business operations analytics is different from traditional analytics.
Business Operational Analytics vs. Traditional Analytics
Advanced analytics in operations focuses on real-time data analysis to optimize day-to-day operations, offering immediate insights for decision-making and process improvements. On the other hand, traditional analytics typically analyzes historical data to identify trends and inform long-term strategic planning. While operational data analysis is action-oriented and time-sensitive, traditional analytics is more strategic.
Here is a brief table highlighting the key differences between operational analytics and traditional analytics:
Aspect | Operational Analytics | Traditional Analytics |
---|---|---|
Purpose | Real-time data analysis for immediate insights | Historical data analysis for understanding past performance |
Speed | Provides almost instant insights | Analyzes data over a longer period |
Level of Detail | Offers detailed, granular insights on current performance | Provides a general overview of past performance |
Use Cases | Supports operational decision-making and real-time adjustments | Used for strategic decision-making based on historical data |
Technology | Utilizes machine learning, AI, and IoT | Employs data warehousing, business intelligence, and data mining |
Why Invest in Operational Analytics: Use Cases and Advantages
The use cases of operational analytics range across various industries, from healthcare, finance, eCommerce, and manufacturing to marketing and customer support. Its applications in business help improve efficiency, decision-making, and business performance. Here are some of the most specific use cases of operational analytics:
Delivering Personalized Offers
Analytics in operations enables businesses to go beyond basic demographic segmentation by analyzing customer preferences, purchasing behavior, and engagement patterns in real time. With these insights, companies can craft highly personalized offers tailored to individual customers.
For instance, in the fashion industry, instead of sending generic promotions, a retailer can use operational analytics solutions to analyze a customer’s preferred styles, sizes, and colors, and then automatically notify them when new items matching those preferences arrive.
Streamlining Agile Development
In software development, operational analytics provides valuable insights into team performance, project progress, resource utilization, and customers’ feedback on product use patterns. Companies can use these insights to improve their products. This data-driven approach ensures continuous improvement in the agile development process, enhancing both productivity and product quality.
Predictive Operational Analytics
In industries such as manufacturing, telecom, and utilities, predictive operational analytics can help foresee equipment failures before they occur. Businesses can schedule timely maintenance by analyzing machine performance data, reducing downtime and repair costs.
For instance, an internet service provider in telecommunications can use operational analytics tools to identify disruptions like downed wires or power outages in real time. By dispatching a repair team and providing estimated fix times proactively, they can address problems before customers complain.
Also Read: 10 Ways Retail Predictive Analytics Drives Growth in Business
Customer Support
Analytics in operations can monitor real-time customer interactions and service metrics, helping businesses identify issues, optimize response times, and improve customer satisfaction through personalized services. For high-value products, customer service representatives can proactively engage clients with a personalized text or phone call, offering a warm welcome and assisting with any setup issues or concerns.
Workforce Management
By analyzing employee performance, shift efficiency, and workload distribution, businesses can optimize workforce allocation based on urgency, impact, and resource availability. It ensures that high-priority projects receive adequate attention while optimizing productivity.
For instance, a retail chain can use these insights to adjust staffing levels during peak shopping hours and redistribute tasks among employees based on their strengths, ensuring a smoother and more efficient workflow across the organization.
Inventory Management
Retailers can use operational analytics to forecast customer demand, track their purchasing patterns, and optimize stock levels. For instance, an eCommerce platform can leverage the power of analytics in operations to predict high-demand periods and manage inventory accordingly. This enables the retailer to prevent stockouts or overstock situations, improving sales performance and customer satisfaction.
Reducing Customer Churn
Operational analytics helps companies predict and prevent customer churn by analyzing usage patterns, service interactions, and feedback data. By identifying at-risk customers early on, businesses can implement targeted retention strategies, such as offering personalized promotions or resolving recurring issues, thereby improving customer loyalty.
For example, a coffee shop could send a customer who hasn’t visited in a while a voucher for a free pie with their next coffee purchase, encouraging them to return and enjoy a complimentary treat.
Enhancing Risk Modeling
In sectors like finance and insurance, operational analytics is critical for developing robust risk models. By analyzing historical data and external factors, companies can predict potential risks, such as credit defaults or fraudulent activities, and make informed decisions to mitigate them. This proactive approach helps reduce financial losses and ensures more accurate risk assessments.
Real-World Examples of Companies Using Operational Analytics
There are several industry leaders who leverage operational intelligence and analytics to transform their extensive datasets into actionable insights, driving personalized customer experiences and proactive maintenance practices. Here are some popular operational analytics examples:
Amazon
Amazon, an eCommerce giant, analyzes customer purchase history and browsing behavior to personalize its marketing strategy. This data-driven approach ensures that each visitor receives tailored product recommendations, enhancing their shopping experience and increasing engagement.
Nissan
Nissan is a leading automotive manufacturer that uses business operations analytics to track the popularity of different car models and colors across various regions. This allows them to adapt their marketing efforts and sales strategy to better align with their customer preferences.
Shell
Shell, a global energy leader, utilizes predictive analytics to monitor the condition of drilling equipment. By forecasting the lifespan of parts and scheduling maintenance before breakdown occurs, Shell reduces operational downtime and minimizes inventory costs.
How to Implement Operational Analytics in Business?
Implementing operational analytics involves a structured approach to ensure that the system aligns with your business goals and delivers actionable insights. Here are the key steps involved in operational analytics implementation process:
Define Clear Objectives
The first step of operational analytics implementation starts from identifying the specific goals you want to achieve with it. Whether it is improving operational efficiency, reducing costs, or enhancing customer satisfaction, having well-defined objectives will pave the way for the entire implementation process.
Establish Relevant KPIs
Identify the key performance indicators (KPIs) that align with your objectives. Ensure these KPIs are directly related to your goals. This step is crucial for ensuring that your operational analytics service is focused and relevant.
Collect and Correct Data
The next step is to gather data from various operational sources, including systems, processes, and external environments, and verify that the collected data is accessible and accurate. This involves ensuring data quality and addressing any gaps or inconsistencies.
Select the Right Tech Stack
Choose the right tech stack and analytics platforms that align with your objectives. These tools should enable real-time data processing, predictive analytics, and easy visualization for actionable insights.
Build an Integrated Analytics Platform
Develop a comprehensive analytics platform that can analyze the collected data, identify patterns, and provide forecasts. The platform should integrate disparate data points to provide a cohesive view, enabling effective operational data analysis and decision-making.
Conduct a Pilot Test
Implement the analytics system on a smaller scale to evaluate its functionalities and effectiveness. The pilot phase will help identify any issues or areas for improvement before a full-scale deployment.
Roll Out the System
After successful testing, you can proceed with a full-scale implementation to integrate the analytics system across the organization, ensuring it meets all IT operations analytics use cases and delivers optimal performance.
Monitor and Maintain
Regularly review the performance of the analytics system to ensure it continues to meet your objectives. Make necessary adjustments based on user feedback and changing business needs to maintain its effectiveness.
Optimizing Business Operations Analytics: Best Practices for Success
To leverage the maximum benefits of operational analytics and ensure it delivers meaningful results, you must implement thoughtful strategies that ensure accuracy, relevance, and actionable insights. The best practices for operational data analytics include:
Align Data with Key Business Goals
Start with the questions your business needs answers to, and collect the data accordingly. Identify whether supplier information, customer data, financial metrics, or transactional insights are most critical. Select the right data model to transform this information into actionable insights that support your goals.
Focus on One Key Use Case Initially
Launch operational analytics by focusing on a specific business issue or performance metric. This controlled start allows for a practical assessment of the system’s capabilities. By narrowing the scope initially, you can optimize the operational analytics solution before introducing it to a broader range of operations.
Tailor Data-Analysis Tools
Ensure the data feeding into your analytics system is accurate and complete. Explore how the platform handles missing values, as many tools generate values based on the data’s contribution to various processes. Regularly review your data models to confirm they deliver meaningful results.
Prioritize Data-Driven Decision-Making
Even with strong analytics, some companies still rely on manual decision-making processes, which can impede their ability to act on insights. Thus, you must shift your organizational culture to prioritize decisions leveled in data analysis to ensure faster, more informed actions.
Enhance Analytical Competence
Develop a strong foundation in analytics by leveraging descriptive analytics and tracking key performance indicators (KPIs). Descriptive analytics reveals past trends, while KPIs help monitor performance over time, allowing for better insight into operational patterns and improvement areas.
Highlight Benefits Across Teams
Operational analytics should be seen as a company-wide initiative rather than a tech-driven project. Thus, you must clearly communicate the operational analytics benefits to all departments, showing how data-driven insights can enhance their daily tasks, streamline decision-making, and improve overall efficiency.
Leverage External Data Sources
Data gaps are inevitable, but external sources can fill those voids. Consider using free datasets, purchasing data, or employing sampling methods to supplement your internal data. External data adds valuable context, minimizes storage needs, and can reduce potential errors in your analysis.
Challenges and Solutions for Operational Analytics Implementation
Even if IT operational analytics use cases and benefits are innumerable, its implementation is not so easy. It presents several challenges that need to be addressed strategically to reap the maximum benefits of operational analytics. Here are some of the most common challenges and their solutions, ensuring the smooth road to IT operational analytics implementation.
Data Silos
Challenge: One of the biggest challenges in operational analytics implementation is dealing with siloed data. When data is scattered across different systems, gaining a holistic view of operations becomes difficult.
Solution: Businesses can overcome this issue by integrating all relevant data sources into a unified platform. They can also rely on data warehousing solutions and cloud-based platforms.
Integration with Legacy Systems
Challenge: Integrating modern operational analytics solutions with older legacy systems can be a time-consuming and resource-intensive process. Legacy systems often lack the flexibility and scalability needed to support advanced analytics.
Solution: Use middleware or API-driven integration solutions to connect legacy systems with modern analytics platforms. Alternatively, you can also migrate your legacy systems to cloud-based infrastructures that are designed to work with advanced data tools.
Resistance to Change
Challenge: Introducing operational analytics can lead to resistance from employees who are accustomed to traditional analytical tools. Teams might hesitate to use advanced, automated insights or fear adopting new tools.
Solution: You should explain the operational analytics benefits to all the departments. Provide training and ensure that analytics tools complement their jobs rather than replace their roles.
Future of Operational Analytics for Businesses
As the volume of big data continues to surge and IoT devices become more embedded in daily operations, the role of operational analytics is experiencing exponential growth. Industry forecasts suggest that the operational analytics market could surpass $127 billion by 2029, rising from $26.8 billion in 2024. This growth is driven by the increasing reliance on smart devices across homes, workplaces, and industrial sectors.
Moreover, as operational analytics services become more accessible and intuitive, we can expect to see increased democratization of data, which helps share the decision-making power across the organization. This trend will empower teams at all levels to leverage the power of data insights in real time, enabling more proactive and agile business strategies.
Organizations that embrace operational analytics for business will experience robust operational reporting, and seamless data integration from multiple sources. These companies will take a collaborative, cross-departmental approach to analytics, ensuring that insights are not siloed but shared across teams for better decision-making.
Unsurprisingly, the future of operational analytics is not just about technology; it is about transforming the way businesses operate and make data-driven decisions at every level.
How Appinventiv Helps You Reach Operational Analytics Excellence
Operational analytics for business relies on high-quality data and advanced technologies to integrate data from different sources and deliver real-time insights. With Appinventiv as your trusted operational data analytics consulting company, you can transform your raw, unstructured data into a powerful asset that propels your business forward, ensuring that every decision is informed and every strategy is optimized for success.
Our team of 1600+ tech specialists is skilled in developing data-driven platforms and integrating advanced analytics tools with existing systems, ensuring seamless data flow and high-quality outputs. We provide advanced operational analytics services that focus on delivering end-to-end solutions, supporting every aspect of your operational strategy, from data collection and management to real-time analysis and reporting.
What’s more? We prioritize scalability, offering you custom operational analytics solutions that grow with your business while delivering continuous improvements. With our deep knowledge of operational data analytics implementation and a proven track record of delivering tailored solutions, we empower businesses to make informed decisions, optimize operations, and gain a competitive edge in today’s data-driven landscape.
So, what are you waiting for? Unlock the power of business operations analytics with us—your partner in achieving operational excellence and sustained business growth.
FAQs
Q. How does operational analytics work?
A. Operational analytics works by collecting and analyzing real-time data from various sources within an organization. This data is processed using advanced technologies such as artificial intelligence and machine learning, providing immediate insights into operational performance. By integrating data from different systems, business operational analytics tools help monitor key metrics, detect anomalies, and drive real-time decision-making to optimize processes and improve efficiency.
Q. What is operational analytics?
A. Operational analytics, a subset of business analytics, focuses on immediate actions. It analyzes real-time data to enhance the efficiency of daily business operations. By using the latest data integration techniques and cutting-edge technologies, IT operational analytics provides businesses with actionable insights, helping them make informed decisions, streamline processes, and quickly respond to changing conditions.
Unlike traditional analytics, operational analytics focuses on real-time data and immediate operational adjustments.
Q. What are the benefits of operational analytics for business?
A. Operational intelligence and analytics offer several advantages to businesses. Some of the most remarkable benefits of operational analytics are:
Enhanced Accuracy with Advanced Technologies: By leveraging the power of advanced technologies like business intelligence, AI, and ML, operational analytics delivers highly accurate and actionable data insights.
Enhanced Data Collection: Operational analytics tools help improve data collection practices. It ensures that all relevant information from different sources is utilized to power decision-making processes.
Improved Collaboration: It promotes better communication and coordination among different departments—including operations, engineering, management, marketing, sales, and executives.
Streamlined Processes: Standardizes and optimizes internal business processes, reducing complexity and improving efficiency across teams with diverse needs.
Effective System Utilization: Business operations analytics helps teams evaluate and enhance the efficiency of their existing systems and processes.
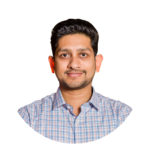
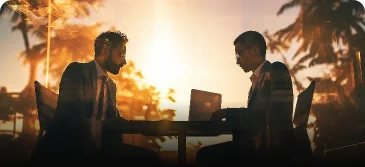
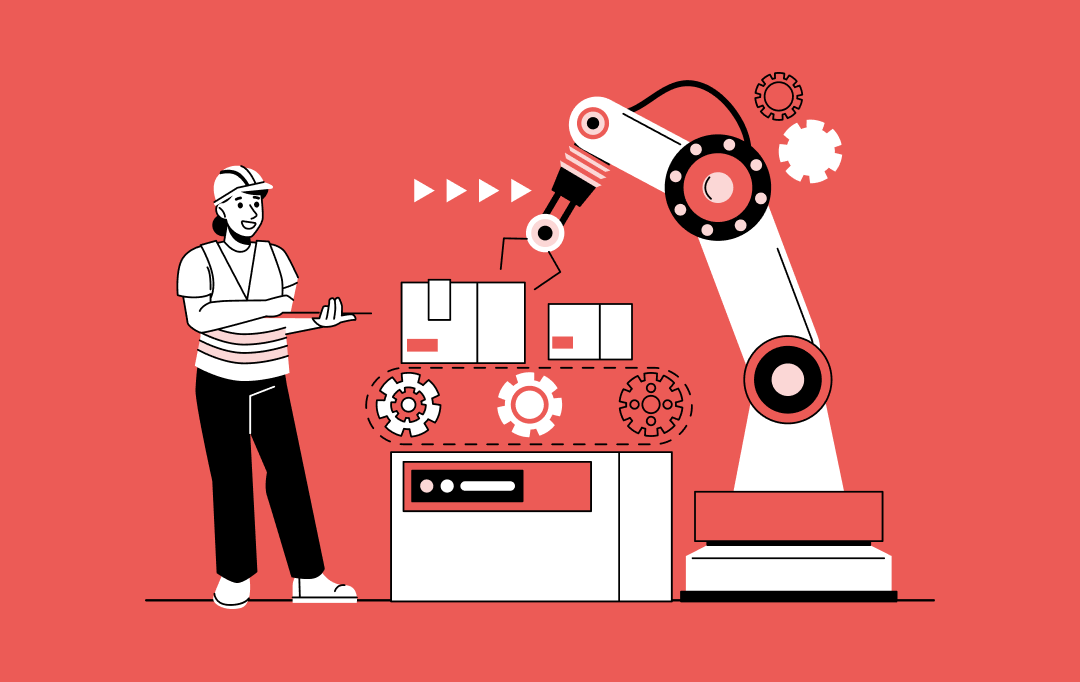
10 Use Cases and Benefits of Predictive Analytics in Manufacturing
Are you trying to stay in today’s manufacturing game with that old-school data analytics approach? Not gonna happen, buddy! Think about that old-school grind: manufacturing plant techies had to hustle around, scribbling notes and logging maintenance records by hand. All that manual intervention and hassle led to nothing more than messy data and bad calls.…
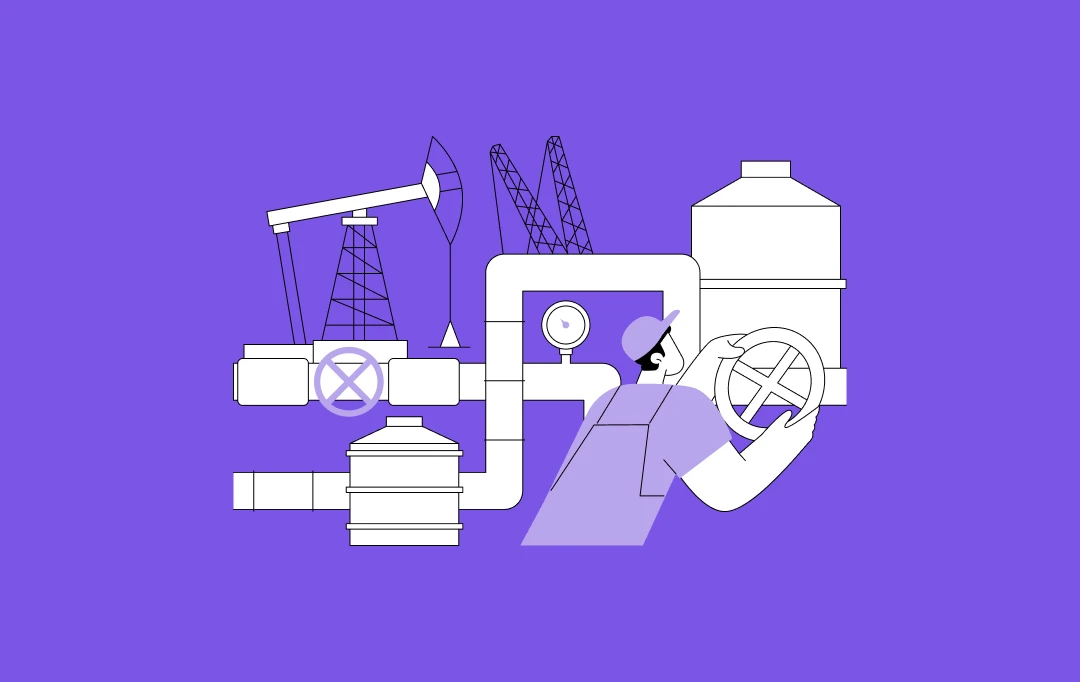
10 Use Cases and Benefits of Adopting Predictive Analytics in the Oil and Gas Industry
The oil and gas industry has always been at the forefront of technological advancements, constantly seeking innovative solutions to improve efficiency and maximize output. It is where predictive analytics emerges as a game-changing solution, transforming how companies manage operations, optimize production, and mitigate risks. In the oil and gas sector, where even the smallest oversight…
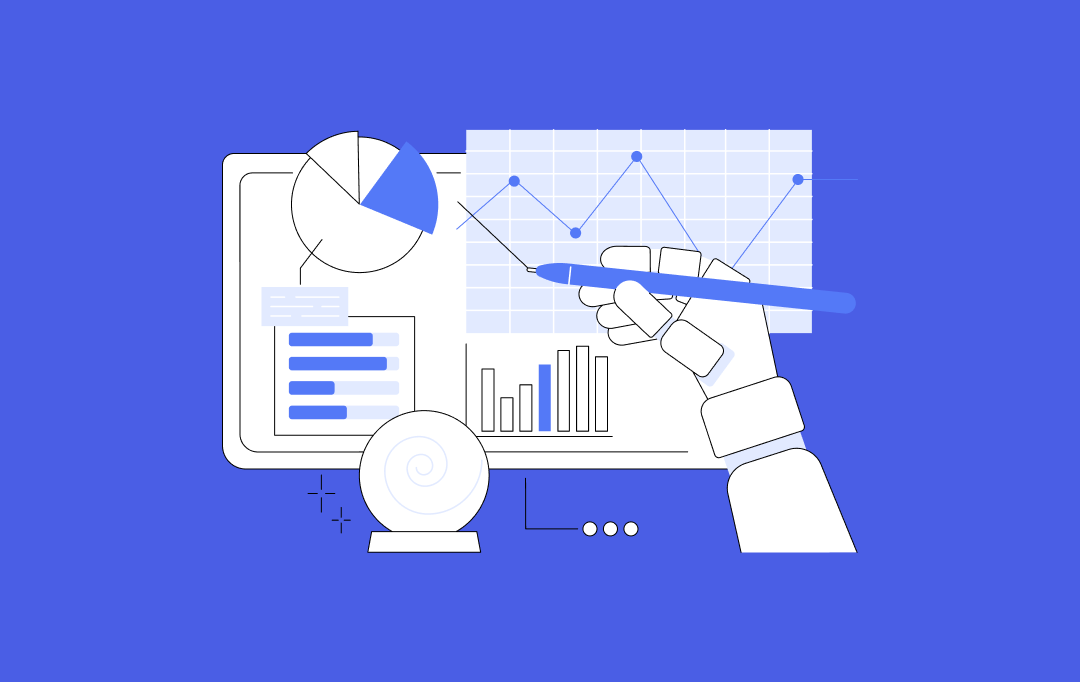
Big Data Analytics in the Oil and Gas Industry - Benefits, Use Cases, Examples, Challenges
Data has become increasingly popular as it drives decision-making across industries, enabling businesses to gain insights and optimize operations. In the era of digital transformation, data generation has skyrocketed, fueled by the proliferation of big data and online interactions. This exponential growth has led to the development of advanced data analytics technologies, empowering organizations to…