- What is Edge AI?
- How does Edge AI work?
- What are the benefits of Edge AI?
- 1. Real-time processing of data
- 2. Better privacy
- 3. Lower internet bandwidth
- 4. Lesser power consumption
- 5. More responsiveness
- What are the use cases of Edge AI?
- Manufacturing
- Retail
- Smart hospitals
- Drones
- Traffic
- Energy
- What is the future of Edge AI?
- What are the challenges of adopting Edge AI?
- 1. Lack of standards in hardware
- 2. Integration with multiple elements
- 3. Limited expertise
In recent years, AI adoption has grown tremendously. With a rise in business data, IoT applications, and customers using more devices than before, it has become sensible for businesses to get the smartness of AI closer to the customers. This is where Edge AI comes into the picture.
As we dive further in the article, we will look into the different aspects of what Edge AI is and the benefits the technology has to offer to enterprises. But first, here’s a peek into the Edge AI market.
What is Edge AI?
Artificial Intelligence depends heavily on the computation of complex machine learning algorithms and data transmissions. Now, what Edge computing does is that it sets up a new age computing approach which moves AI closer to where data generation and its computation takes place. This merger of AI and edge computing has given birth to a new domain known as Edge computing AI.
The technology enables creation of faster insights and computing, greater security and better control over operations. The result of this is that it helps create performance-heavy AI apps while keeping the operation prices down.
What’s best about this technology is that it powers autonomous adoption of deep learning processes, machine learning, and brings advanced algorithms on the Internet of Things (IoT) devices itself, all away from the cloud services. However, with a reliance on cloud, is there any difference in cloud computing and Edge AI architecture?
Cloud computing and Edge AI are usually not interchangeable as they both come with individual applications and strengths. Edge AI architecture is brought to use when you handle time-sensitive data, perform processes in remote areas that need local storage, and operate smart devices. The limitations consist of high computation power, restrictions of the deep learning frameworks, and the presence of multiple inference hardware.
Cloud computing, on the other hand, enables remote on-device processing with huge computation power over the cloud. While the cloud offers more design and architecture options, it lowers the power consumption needed for high-level processing.
How does Edge AI work?
For a machine to view, detect objects, understand speech, drive cars, or copy other human skills, they will have to imitate human intelligence. This is where AI comes in. Artificial Intelligence uses a data structure known as deep neural network which copies cognition. These are trained for answering specific questions by being fed with different versions of the questions and their answers.
The training process, which is also called “deep learning,” operates within a data center because of the massive amount of data needed to train the model. Once the training is complete, the algorithm becomes an “inference engine” which can answer questions.
In the case of Edge AI deployments, this inference engine operates on devices in varied locations like hospitals, cars, factories, home, and satellites. Once AI looks at a problem, the data is uploaded to the cloud for training, which replaces inference engine. This loop carries a significant impact in bettering the model performance; once the Edge AI models get deployed, they get smarter.
What are the benefits of Edge AI?
There are a range of advantages that come associated with Edge computing AI. Now, irrespective of what those are, they all gravitate towards better processes and customer experience.
1. Real-time processing of data
The biggest benefits of Edge AI is that the technology brings in high-performance computation power to the edge where the IoT devices and sensors are based.
AI edge computing technology enables adding AI use cases directly on the field devices. The most common Edge AI examples can be seen in how the software can process data and machine learning with the help of deep learning algorithms in the autonomous Edge AI applications, like autonomous vehicles.
When incorporated in an autonomous vehicle, the technology can process data in a few milliseconds, preventing accidents in real-time.
2. Better privacy
In case of Edge AI, the data processing activities are performed at a local ground on the edge computer. Because of this, less data gets sent to the cloud which lowers the risk of mishandling of data or getting misappropriated.
Now, since the data is gathered and processed near to the devices, less transmission happens which leads to better data security.
3. Lower internet bandwidth
Since the Edge computing AI operates with data processing happening locally, businesses get to save a huge amount of money on the internet bandwidth since fewer data gets transmitted via the internet.
If you use Amazon AWS AI Services for your business needs, you would know how costly it can get to perform AI processes in the cloud. With Edge AI, cloud can get reserved as only a repository for the post-processed data needed for analysis.
4. Lesser power consumption
With Edge AI solutions because the data gets processed at a local stage, businesses get to save a lot on the energy costs as they don’t necessarily have to remain connected to the cloud for transferring data back and forth between edge platform and cloud. Moreover, a majority of the edge computing devices come with power consumption and efficiency features.
What is important to consider here is that because a majority of edge apps are deployed in remote environments, it is necessary for the edge computers to balance the performance and power.
5. More responsiveness
Edge AI technology tends to process the data locally, making it a lot more responsive compared to cloud computing where the device collects data, sends it to the cloud for getting processed, and waits for it to be sent back.
All of this happens in millisecond processing time. This leads to Edge AI solutions taking expedited actions and making faster decisions. This results in apps which need instantaneous feedback, like intelligent automation, autonomous vehicles, and robotics.
What are the use cases of Edge AI?
Edge AI solutions emerged as the result of the merger of artificial intelligence and edge computing. This combination is engineered to bring the facilities of deep learning artificial intelligence algorithms closer to the surface. The adoption of Edge AI applications can be seen across a range of industries and use cases. Let us look at some of the top Edge AI examples.
Manufacturing
Factories which work with precision manufacturing are needed to ensure that there is complete safety and accuracy in the product. Now, when you add in Edge AI in the mix, you get to ensure that the factory floor is efficient and safe. Through the inclusion of machine vision, you can monitor the product quality with amazing precision. It also helps with product automation and prediction of mechanical failures.
Procter & Gamble uses the technology in the way of inspection cameras. It prevents imperfections from getting out of the factory, by analyzing the videos collected from the floor cameras.
Retail
There is rarely a retail house which is missing out on customer analytics. However, at the core of it, customer analytics is majorly dependent on everything digital – their views, where they left the website, when did they purchase what, etc. For the brick and mortar businesses, this analytics is usually limited to receipts.
Edge AI changes that through video analytics. By bringing the computational power near the stores, it is able to extract key data from store videos around how happy the visitors are, what are they looking for, what are they giving importance to – color, price, size, touch, etc.
Smart hospitals
The adoption of edge computing and artificial intelligence in the medicine domain would help aid and promote patient care while enhancing the operational efficiency.
Edge AI apps help with better data security which is necessary for the hospitals to function better. Medical industries can adopt Edge AI for performing tasks such as –
- high precision thermal screening
- inventory management
- remote monitoring of patients
- prediction of ailments
Drones
The use cases of Edge AI in drones are seen across a range of events like construction, traffic monitoring, and cartography. Drones work on visual search, image recognition, and object identification, tracking. When AI is added to the technology, it is able to give sense to the data which is gathered by imitating human search behavior.
The application of Edge AI in drones would enable the data to get analyzed effectively. It will also aid tracking in real-time, predictive maintenance, object recognition, and face recognition.
Traffic
Edge AI has a massive use case in the transport and traffic domain. For example, air crafts and autonomous ships generate a lot of data which when analyzed correctly and speedily can improve safety. Another example of the technology can be seen in the technology helping with calculating passengers’ numbers and locating the nearest vehicle with utmost accuracy.
Energy
The energy space has established how a smart grid can produce a vast amount of data. And not just that. A smart grid can power demand elasticity, monitor consumption, properly utilize renewable energy, and even decentralize energy production. Achieving all of this, however, would require the grids to communicate between devices, which would get delayed when the data is getting transferred between the device and a traditional cloud service. This is where Edge AI proves handy.
So here were the top use cases or Edge artificial intelligence applications. However, these are only the surface level applications of the technology, the reality is that it has a place in every use case where AI can be adopted.
Now that we have looked into the basics of what is Edge AI and what are its use cases an AI software development company works around, the primer would remain incomplete without looking into the challenges that come attached with the technology. We will get into that just as soon as we cover ‘what to expect’ from Edge AI.
What is the future of Edge AI?
The growth in Edge AI is on an obvious rise. However, this is only the beginning. There are a number of trends which have been shaping up in the domain. Let us look into them.
Management of Edge AI will be an IT task. Even though Edge AI is on a rise, their deployment remains challenging. In order to move to the production stage, the technology will be managed by the IT department. They can be seen as the right point of contact when it comes to managing, security, and scalability of the model.
Convergence of Edge AI and IIoT. When it comes to AI adoption, manufacturing industries, especially ones that have incorporated IoT, emerge as the biggest name in the domain. In the years to come, we are poised to see the merger of IIoT and Edge AI in use cases around sensors and cameras for inspection, preventive, predictive maintenance.
Rise in Edge data centers. By 2024, more than five million servers are going to be deployed at the edge. These data centers would only rise in number because of a range of factors like:
- 5G network
- IoT proliferation
- SDN, and NFV tech
- Video streaming with AR and VR
The demand is only going to rise on the back of facilities like lower latency, intermittent connectivity issues, and having data storage closer to the end users.
What are the challenges of adopting Edge AI?
Although the implementation of Edge AI is very advantageous, it comes with challenges. There are a number of elements which make the technology difficult to implement.
1. Lack of standards in hardware
Edge computing comes with a massive reliance on the hardware. What makes it worse is that Edge AI hardware which are available in the market today do not have any standardized units. Moreover, there are a number of elements such as use cases, power consumption, memory needs, processors, etc. which have to be considered.
2. Integration with multiple elements
Hardware is one element of the AI model. It is not uncommon for developers to use multiple models and frameworks to create applications. This integration, however, can be challenging. Additionally, businesses might also make use of third-party platforms that would need fresh integration with the software and hardware used for Edge artificial intelligence.
3. Limited expertise
Edge AI applications are constantly evolving and so is every industry it is getting adopted into. Keeping up with this demand calls for having expertise across all the latest hardware selection, integration of tools, optimization of deployment and testing model to deploying and testing, etc. Finding a team of people who have the expertise of not just Edge AI but also the changing tech stack can be a challenge.
Solving these challenges requires a skilled team who has expertise in working with Edge devices and a plethora of Edge artificial intelligence use cases across industries, including generative AI services. Well, you don’t have to look very far. Appinventiv has helped over 25+ businesses across industries explore the power of Edge AI and use them across different use cases. Want to discuss your idea? Get in touch with our team of AI experts today!
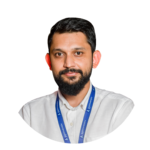
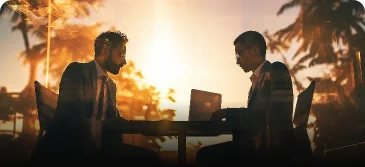
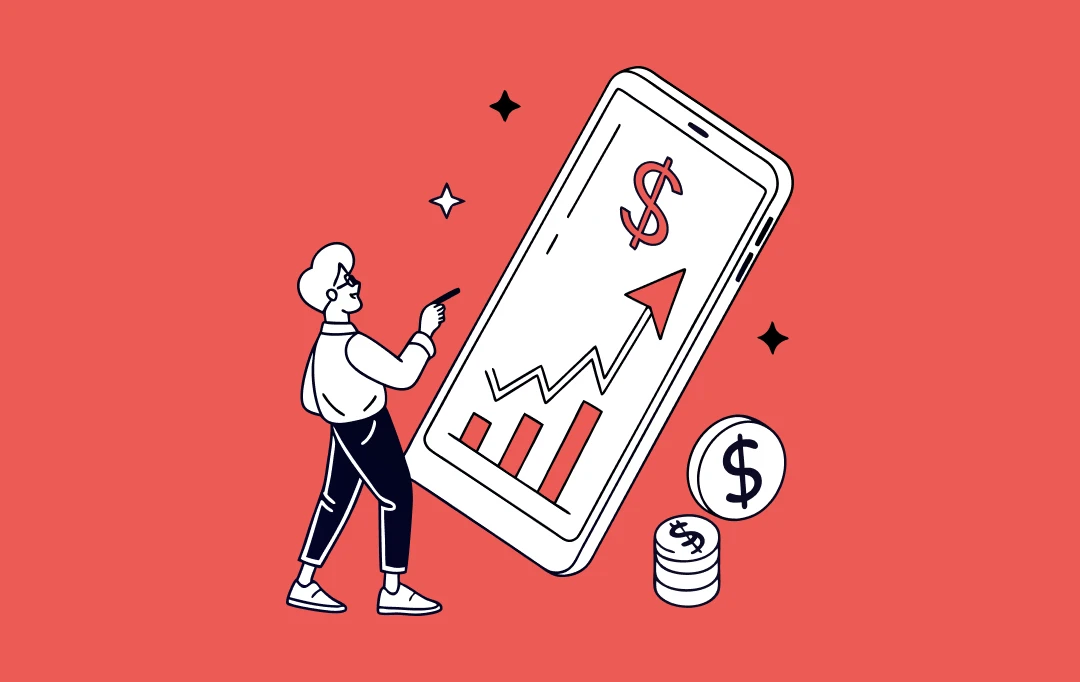
How Much Does It Cost to Build an AI Trading App Like Moomoo?
Trading apps have undoubtedly solved the major pain point of the public - eliminating middlemen from investing their money, Which keeps them in fear that they might get cheated or lose their money. However, trading apps have facilitated users with the transparency to perform trading safely and swiftly. In the era of smartphones and AI,…
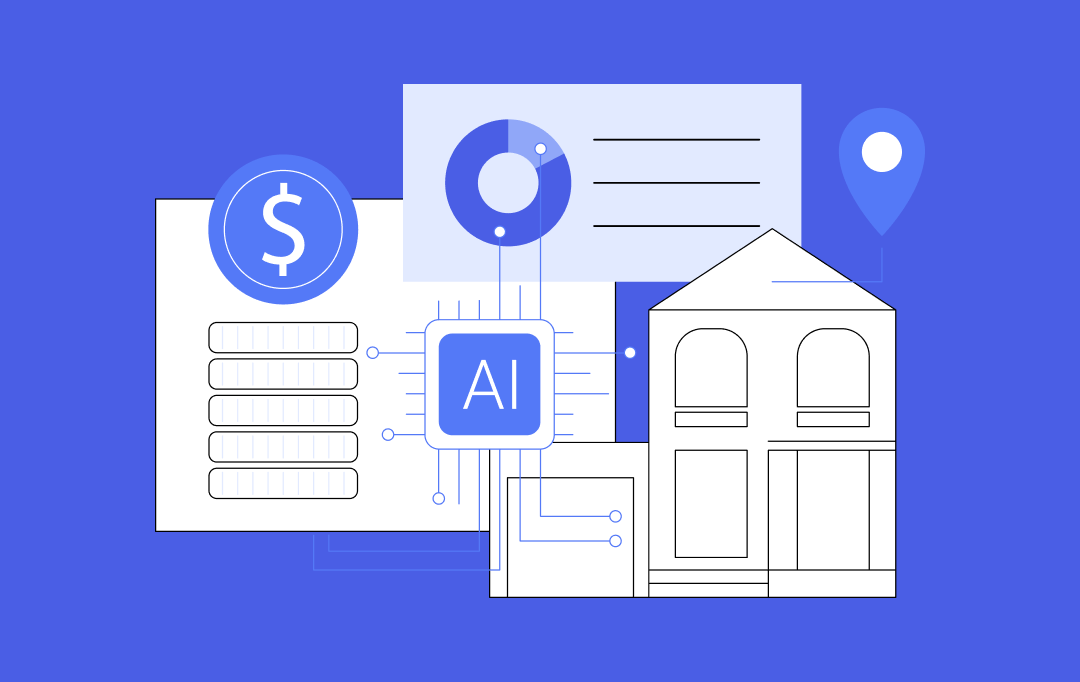
15 Explorative Use Cases of AI in Mortgage Lending
In an era where technological advancements are revolutionizing every sector, mortgage lending has been slow to embrace change. The industry has been bogged down by outdated processes, increasing operational costs, and regulatory pressures. However, with the introduction of AI in mortgage lending industry, a shift is occurring that promises to address these pain points while…
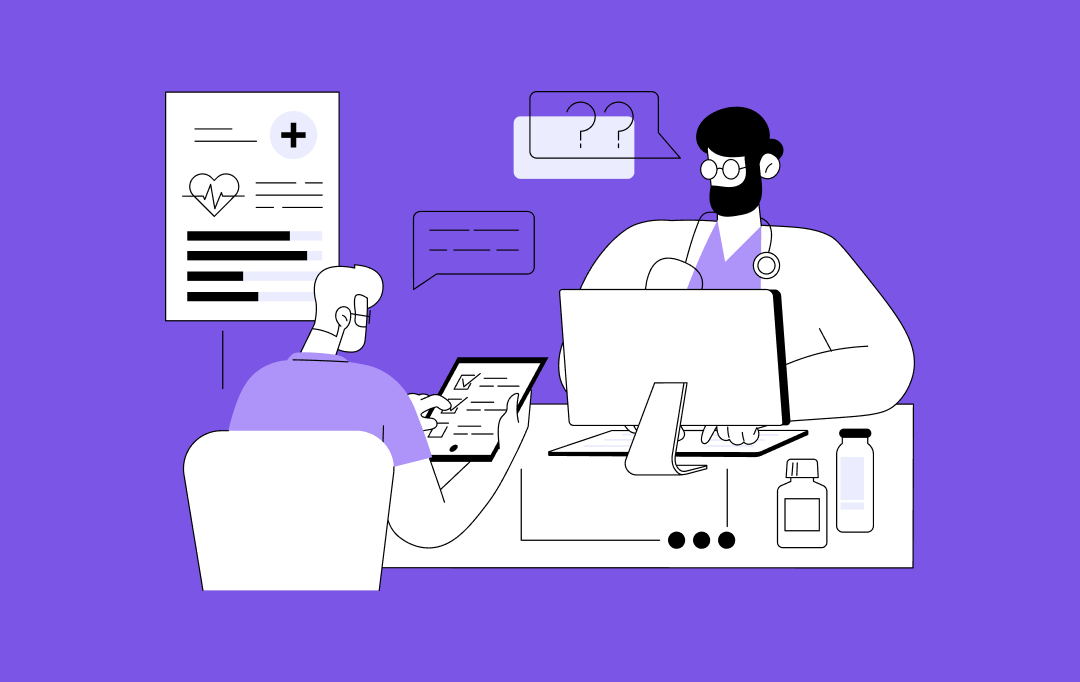
How to Develop AI Medical Transcription Software? Costs, Process, and Benefits
Developing accurate and efficient medical transcriptions manually has always been a painstaking process, fraught with many challenges. Manual transcription often leads to errors, misinterpretations, delayed patient care, and the high costs associated with hiring skilled professionals. As the volume of medical data grows, the pressure to maintain accuracy without compromising efficiency intensifies. It's time to…