- What is a Neural Network and How Does it Work: Understanding its Business Value
- A Glimpse into the Business Value of Neural Networks
- Industry-Wise Business Applications of Neural Networks
- Finance
- Healthcare
- Retail
- Manufacturing
- Transportation
- Telecommunications
- Energy
- Agriculture
- Challenges of Artificial Neural Network Integration in Businesses
- Data Quality and Quantity
- Computational Resources
- Technical Expertise
- Integration with Existing Systems
- Ethical and Privacy Concerns
- Ongoing Maintenance and Updating
- Leveraging Neural Networks with Appinventiv
- FAQs
As we approach the end of 2024, it’s clear that artificial intelligence (AI) has evolved from being a mere hype to a pivotal force that has been the backbone of major innovations driving businesses across multiple industries. Among the many AI technologies, neural networks stand out due to their ability to mirror the complex network of the human brain, enabling machines to learn and think based on past experiences.
This unique capability has revolutionized the approach to complex problem-solving, allowing businesses to analyze and interpret massive volumes of data quickly. This helps improve the overall existing processes and paves the way for innovative business models and strategies that are smarter and more efficient.
According to a report from Allied Market Research, the global artificial neural network (ANN) market was valued at $227.8 million in 2022 and is expected to reach a valuation of $1.4 billion by 2032, witnessing a CAGR of 19.9% from 2023 to 2032. This growing market size can be attributed to the increasing adoption of cloud-based solutions and big data technologies, along with the rising demand for predictive solutions and personalized AI-driven services across diverse sectors such as finance, healthcare, and retail.
This exponential growth showcases the significant role neural networks play in driving operational efficiencies and strategic innovations. Businesses across the globe are leveraging ANNs to refine customer service and optimize supply chains, as well as develop solutions that address previously unsolvable challenges. From improving medical diagnostics with advanced imaging to facilitating quick financial decisions, the business applications of artificial neural networks are foundational to hundreds of digital transformations.
Thus, if you are a business navigating this fast-evolving tech landscape, effectively using neural networks could be crucial for staying competitive and improving customer satisfaction. This blog will explore how various industries are adopting the applications of neural networks to reshape their operations, providing insights into real-world examples and the potential future of neural networks.
What is a Neural Network and How Does it Work: Understanding its Business Value
A neural network is a collection of algorithms that works to identify patterns within data by imitating how the human brain functions. Simply put, it is a sophisticated data modeling tool that can help businesses make sense of complex patterns and data.
The artificial neural network architecture is made up of layers of nodes, or neurons, each processing incoming data and sending it to the next layer. The first layer handles the initial data, similar to how our senses perceive the world around us. As the data moves through each layer, it is refined and transformed, extracting more detailed features at each step. The final layer then produces the output.
What makes neural networks in artificial intelligence especially effective is their ability to learn from results. They adjust the connections between nodes based on the accuracy of previous outputs, allowing them to improve their performance over time without being explicitly programmed to solve specific tasks.
Here’s a brief explanation of some common types of artificial neural networks used in business:
1. Feed-forward Neural Networks (FNN): This is the simplest type of neural network. Here data moves in one direction from input to output and is ideal for pattern recognition tasks.
2. Recurrent Neural Networks (RNN): These are networks with loops that allow them to process sequences of data. RNNs in comparison to transformers are useful for time series analysis and natural language processing.
3. Convolutional Neural Networks (CNN): These are designed for grid-like data such as images and use convolution operations to capture spatial hierarchies.
4. Autoencoders: These neural networks learn efficient representations of data and are commonly used for dimensionality reduction and feature learning.
5. Generative Adversarial Networks (GANs): They consist of two networks competing against each other to generate new data instances. They are useful for creating realistic images and models.
A Glimpse into the Business Value of Neural Networks
Predictive Analytics: Neural networks excel in identifying patterns and predicting future trends. This is invaluable for sectors like finance and retail, where forecasting accuracy can significantly influence strategic decisions.
Also Read: A Comprehensive Guide on using Predictive Analytics for Mobile Apps
Improved Customer Insights: By analyzing customer data, neural networks in AI solutions can help businesses personalize services and predict customer needs. These applications of neural networks lead to better customer engagement and satisfaction.
Enhanced Decision-Making: Neural networks improve decision-making processes by providing accurate predictions and analyses based on historical data. This helps businesses make informed decisions quickly, reducing risks and maximizing opportunities.
Automation of Repetitive Tasks: One of the most vital advantages of artificial neural networks is automation of repetitive and time-consuming tasks. This helps in increasing efficiency and allowing employees to focus on more creative and strategic activities.
Risk Assessment: They excel in assessing risks by analyzing complex datasets to identify potential issues before they become problematic. This capability is crucial for proactive management and maintaining a competitive edge.
Innovation Acceleration: Neural networks accelerate innovation by enabling the rapid prototyping of ideas using simulations. This reduces the overall time and cost associated with traditional R&D.
Dynamic Learning Capabilities: These networks continuously learn and adapt from new data, improving their algorithms over time without human intervention. This dynamic learning capability ensures that the systems remain effective as business environments evolve.
Scalability: Neural networks in AI software can handle increasing amounts of data and more complex decision environments as businesses grow. This makes them scalable solutions that do not require constant redesign or significant additional investments.
We hope these benefits of artificial neural networks have helped you understand how you can leverage them to streamline operations, innovate faster, and stay ahead in competitive markets, ensuring sustainable growth and success.
If you are still a bit wary of the benefits and business applications of artificial neural networks, well let us offer you a quick insight into the latest findings on power of ANNs. A doctor from the University of Alabama in Huntsville has received a $1 million Air Force grant to develop artificial neural networks for combating RF spoofing, a significant threat in communication networks. This initiative will utilize Generative Adversarial Networks (GANs) to enhance defense mechanisms by simulating realistic RF scenarios to detect and mitigate spoofing activities effectively. The project aims to bolster both national security and the reliability of global communication systems.
Simply put, this project on using artificial neural networks to combat RF spoofing shows how ANNs can significantly boost business security. By integrating ANNs, companies can protect their data and communications from advanced cyber threats, enhancing security and building trust with stakeholders. This proactive approach to cybersecurity can set businesses apart, providing a crucial advantage in managing emerging risks. Let us now move ahead and look at the multiple applications of neural networks in detail below.
Industry-Wise Business Applications of Neural Networks
AI Neural networks are revolutionizing various industries by automating complex decision-making processes and enhancing operational efficiency. Here’s how these powerful AI tools are being applied across different sectors to drive innovation and streamline workflows.
Finance
Neural networks in finance are specifically used for fraud detection, risk management, and algorithmic trading. They can analyze vast volumes of transactions to identify unusual patterns that may indicate fraudulent activity. In addition to this, they can assess credit risk and automate trading decisions by predicting market trends based on historical data.
For example, JPMorgan Chase is one of the famous examples of neural network business applications that uses the said technology to detect fraudulent transactions and for risk management, helping to enhance security and decision-making in financial operations.
Also Read: The Transformative Impact of AI in Finance: Key Use Cases
Healthcare
In healthcare, neural networks contribute significantly to diagnostic processes, patient monitoring, and personalized medicine. They process medical imaging to detect and diagnose diseases early and accurately. Furthermore, they also help in analyzing patient data to predict health risks and outcomes. This enables healthcare providers to tailor treatments to individual patients.
For instance, Google Health using ANNs is one of the powerful examples of neural networks in healthcare. It uses deep learning technology for enhancing the accuracy of medical imaging analyses, like identifying signs of diabetic retinopathy or anemia in eye scans.
Also Read: How AI is transforming the healthcare industry
Retail
Retail industry has several neural networks use cases that businesses can exploit to gain a comparative edge. AI Neural networks enhance customer experiences and streamline inventory management in the retail sector. They can help in predicting the purchasing trends, optimizing the stock levels, and personalizing the marketing strategies by analyzing customer behavior data. This leads to more effective marketing campaigns and a reduction in stock shortages or surpluses.
For instance, Amazon and Aliexpress are few of the best examples of the business applications of artificial neural networks. They use neural networks to provide product recommendations and optimize inventory management based on real-time consumer behavior analytics.
Manufacturing
In manufacturing, AI neural networks are used for predictive maintenance and quality control. They predict when machines are likely to fail, paving the way for timely maintenance. They also inspect products for defects during manufacturing, ensuring high quality without slowing down production.
For example, General Electric (GE) leverages neural network technology to predict when industrial equipment needs maintenance, thereby reducing downtime and maintaining production efficiency.
Also Read: How AI is proving as a game changer in the manufacturing industry
Transportation
In the transportation industry, neural networks optimize routing and logistics operations. They analyze traffic data in real-time to optimize delivery routes, reducing delivery times and fuel consumption. In addition to this, they play a crucial role in autonomous vehicle technology, processing data from vehicle sensors to make driving decisions.
For example, Tesla uses neural networks to improve its Autopilot system’s decision-making capabilities, enhancing its vehicles’ safety and efficiency.
Telecommunications
Neural networks in telecommunications are used for network management and customer service. They predict network failures, analyze traffic to prevent bottlenecks and enhance customer interaction through automated systems that handle queries and complaints.
For instance, AT&T, being a dedicated example of business applications of artificial neural networks, uses the technology to monitor network conditions and predict potential downtimes, which helps in proactive maintenance and ensuring uninterrupted service.
Energy
In the energy sector, neural networks forecast demand and optimize energy distribution. They predict energy consumption patterns and adjust supply dynamically, improving efficiency and reducing waste. They also detect anomalies in energy infrastructure, preventing potential failures.
For example, Shell uses neural networks to dynamically balance energy supply and demand, improving the efficiency of its operations and reducing unnecessary energy wastage.
Also Read: AI in the Energy Sector: Significance, Use Cases, Examples
Agriculture
Neural networks in agriculture help in crop monitoring and yield prediction. They analyze images from drones or satellites to assess crop health, predict yields, and detect pests and diseases early. This helps in making informed decisions about irrigation, fertilization, and pest control.
For instance, John Deere uses neural network-based systems to analyze aerial imagery to detect early signs of disease or distress in crops. This paves the way for timely interventions to protect yields.
After looking into the multiple business applications of artificial neural networks, let’s now move ahead and look at the integration challenges of artificial neural networks that businesses must understand in order to seamlessly move forward towards their digital prowess.
Challenges of Artificial Neural Network Integration in Businesses
Integrating Artificial Neural Networks into businesses can lead to significant improvements in efficiency and insights. However, implementing these systems comes with its own set of challenges. Let’s discuss some common challenges businesses face when deploying AI and neural networks in business applications and explore their potential solutions.
Data Quality and Quantity
Artificial Neural Networks (ANNs) need a lot of accurate and complete data to train properly. Many businesses find their data is either incomplete, inconsistent or spread out across different areas of the organization. This issue makes it difficult for ANNs to learn effectively and produce reliable results.
Implementing comprehensive data governance practices can help improve data quality in the solutions powered by neural networks in AI. Businesses should invest in data-cleaning processes and integration technologies that consolidate data sources. This ensures the neural network has access to reliable and comprehensive training data.
Computational Resources
Training ANNs is a complex process that requires intensive resources and demands substantial computational power and storage. This requirement can lead to high costs and complex management issues, especially for businesses without existing robust IT infrastructure.
Adopting cloud computing can effectively address these challenges. Cloud services provide scalable computational resources, allowing businesses to adjust their processing power and storage based on immediate needs. This scalability helps manage costs efficiently and eliminates the need for large initial investments in hardware.
Technical Expertise
Developing and maintaining neural networks in business applications requires a deep understanding of machine learning and data science expertise, which is not readily available in many organizations. This lack of specialized knowledge related to AI and neural networks can affect the use and ongoing management of these models.
To overcome this, businesses can partner with AI service providers that can quickly bridge the knowledge gap, enabling the deployment and maintenance of ANNs. They can further help businesses understand the importance of neural networks while providing ongoing support and training to ensure that their teams are well-equipped to utilize these technologies effectively.
Integration with Existing Systems
Integrating neural networks in artificial intelligence with existing IT infrastructure and business processes can pose significant challenges. The complexity of these systems can lead to disruptions and require substantial modifications to current business operations.
Implementing a phased integration strategy can help minimize these disruptions associated with the implementation of neural network business applications. Businesses should start with pilot projects in non-critical areas, making way for them to refine their approach before rolling out ANNs on a larger scale. This can help in ensuring smoother integration.
Ethical and Privacy Concerns
The deployment of ANNs raises several ethical concerns, including privacy issues and potential biases in decision-making. These concerns can impact customer trust and compliance with regulations.
Establishing clear guidelines on AI ethics and conducting regular audits are vital steps in ensuring the responsible use of neural networks. In addition, employing techniques to reduce decision-making biases and enhance the transparency of AI systems can further align ANN deployments with ethical standards.
Also Read: Beneath the Code: Dissecting AI’s Fundamental Risk and their Countermeasures
Ongoing Maintenance and Updating
Neural networks require continuous updates and maintenance to remain effective as business environments evolve and new data becomes available. Without regular updates, the performance of ANNs can degrade over time.
Setting up regular maintenance schedules for retraining and updating ANN models is crucial. Automated monitoring tools can assist in detecting when models start to underperform. This ensures timely updates and maintains the efficacy of the neural networks in business applications.
Leveraging Neural Networks with Appinventiv
We hope our blog has made you seamlessly understand the various business applications of artificial neural networks. As businesses look to harness the power of Artificial Neural Networks (ANNs) to drive innovation and efficiency, partnering with the right technology provider is crucial.
As a leading AI development company, we can help you integrate ANNs seamlessly into your business operations. Our expertise in developing and deploying cutting-edge AI solutions positions us uniquely to help businesses capitalize on the transformative potential of neural networks.
Our team consists of multiple seasoned experts in machine learning and neural networks, including data scientists and AI specialists who are well-versed in the latest advancements in AI technologies. Our professionals have a proven track record of delivering robust AI solutions across various industries, ensuring that our clients benefit from tailored, high-impact strategies.
We specialize in artificial neural network development, leveraging our deep expertise to craft tailored AI solutions that drive business innovation and efficiency. Whether it’s streamlining operations, enhancing customer engagement, or driving product innovations, Appinventiv ensures that neural network applications are precisely created to deliver measurable results.
Some of our well-known AI-powered projects include Mudra, Vyrb, YouCOMM, JobGet, AI in banking, Chat & More, Gurushala, etc. These projects showcase our ability to implement AI across various sectors, demonstrating our broad expertise and commitment to innovation.
Let us help you unlock the full potential of artificial neural network applications to transform your business operations and achieve new heights of innovation and efficiency.
FAQs
Q. What is an artificial neural network?
A. An artificial neural network is a computer system modeled on the human brain. It uses a network of interconnected nodes, or neurons, to recognize patterns and solve complex problems. Some of the vital neural network business applications include the likes of speech recognition, image analysis, and predictive modeling and their ability to handle complex pattern recognition.
Q. Why do businesses use neural networks?
A. Businesses use neural networks because they can process large and complex data sets that traditional computing can’t easily handle. They are adept at recognizing patterns and making accurate predictions based on those patterns, learning and improving over time. This capability makes them indispensable for tasks such as optimizing supply chains, personalizing customer experiences, and automating decision-making processes.
Q. What are some of the business applications of neural networks in artificial intelligence and machine learning?
A. Artificial neural networks are a specialized branch of machine learning designed to mimic the human brain’s function. While general machine learning techniques handle tasks like data analysis and pattern recognition, neural networks excel at tasks that require complex problem-solving and decision-making that resembles human thinking.
These networks are integral to enhancing computational models and are particularly capable of handling tasks like interpreting large data sets, automating decision-making processes, and identifying subtle patterns, all of which are essential for advancing strategic business initiatives. Companies leverage neural networks in artificial intelligence in areas such as customer behavior analysis, financial forecasting, and operational optimization, harnessing their power to innovate and maintain competitive advantages in their respective industries.
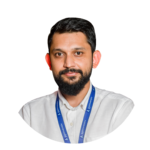
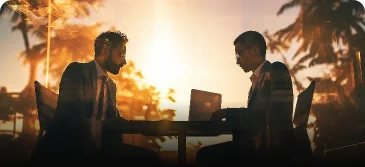
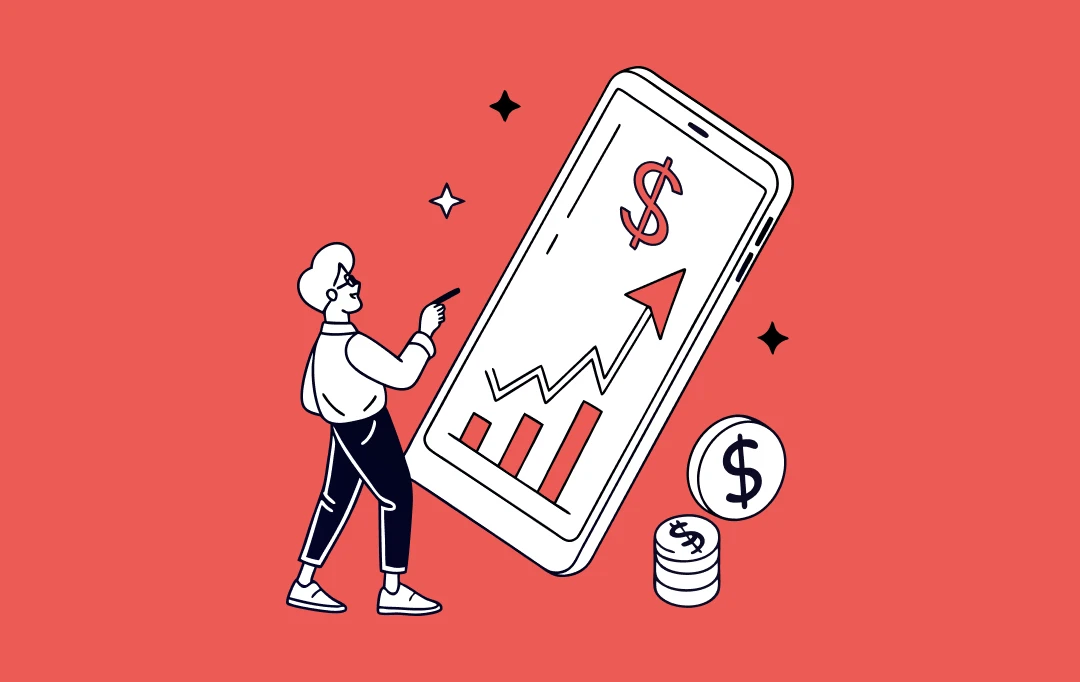
How Much Does It Cost to Build an AI Trading App Like Moomoo?
Trading apps have undoubtedly solved the major pain point of the public - eliminating middlemen from investing their money, Which keeps them in fear that they might get cheated or lose their money. However, trading apps have facilitated users with the transparency to perform trading safely and swiftly. In the era of smartphones and AI,…
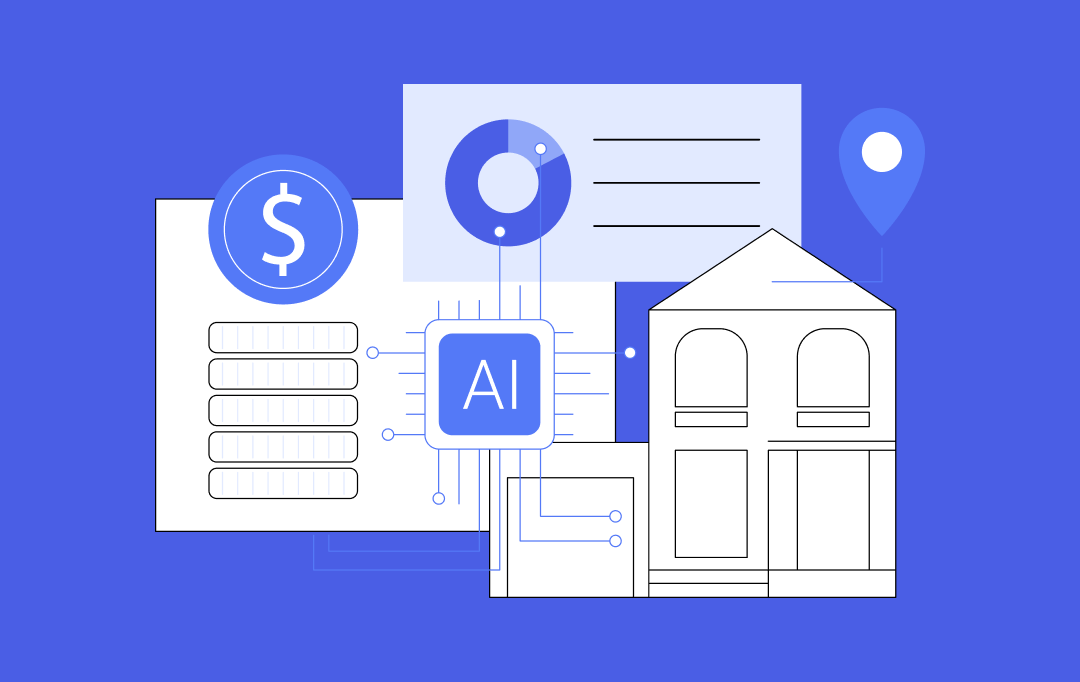
15 Explorative Use Cases of AI in Mortgage Lending
In an era where technological advancements are revolutionizing every sector, mortgage lending has been slow to embrace change. The industry has been bogged down by outdated processes, increasing operational costs, and regulatory pressures. However, with the introduction of AI in mortgage lending industry, a shift is occurring that promises to address these pain points while…
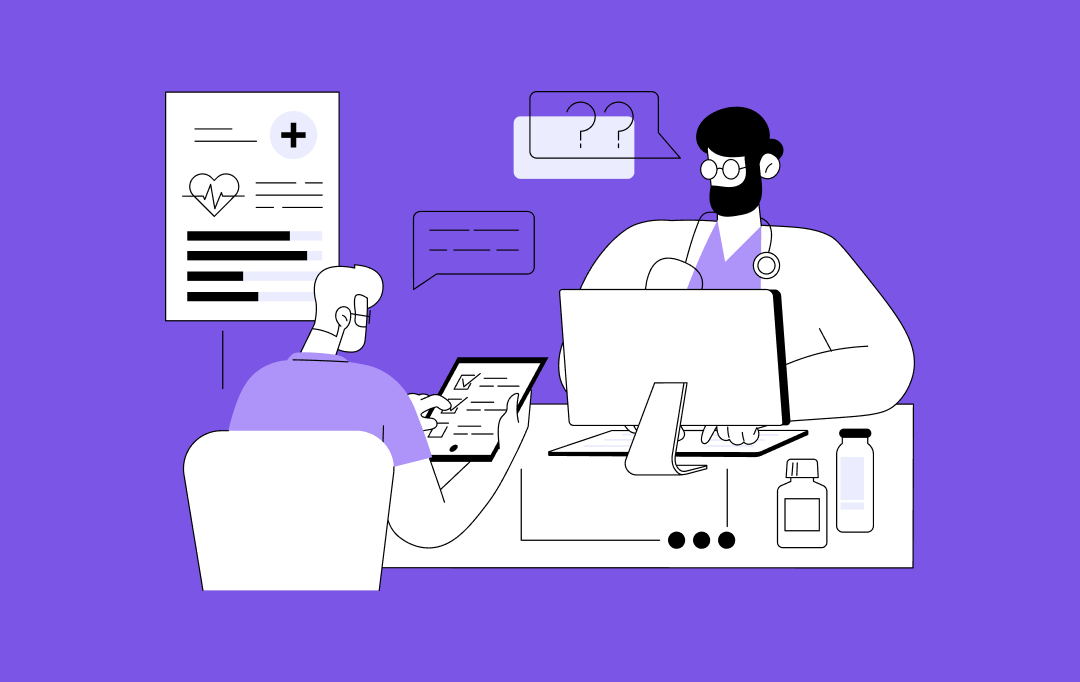
How to Develop AI Medical Transcription Software? Costs, Process, and Benefits
Developing accurate and efficient medical transcriptions manually has always been a painstaking process, fraught with many challenges. Manual transcription often leads to errors, misinterpretations, delayed patient care, and the high costs associated with hiring skilled professionals. As the volume of medical data grows, the pressure to maintain accuracy without compromising efficiency intensifies. It's time to…